Traditional cybersecurity methods are falling short as cyber threats continue to evolve. Artificial intelligence (AI) and machine learning (ML) are revolutionizing threat detection through automated analysis of vast data sets, real-time identification of anomalies, and predictive capabilities to preempt attacks.
The digital environment is changing at a fast pace, and cyber threats are becoming more complex and destructive. Conventional methods of cybersecurity are becoming less and less efficient against advanced techniques such as social engineering, zero-day exploits, and advanced persistent threats (APTs). Organizations need to incorporate more sophisticated threat detection capabilities in order to detect and thwart the threats before they can cause damage.
Artificial Intelligence (AI) and Machine Learning (ML) provide necessary mechanisms to boost cybersecurity because they have the capability of automating the threat detection and response processes. AI and ML algorithms scan large volumes of data very fast making it possible to identify patterns and anomalies that may indicate a cyberthreat and, therefore, immediate detection and neutralization of these threats.
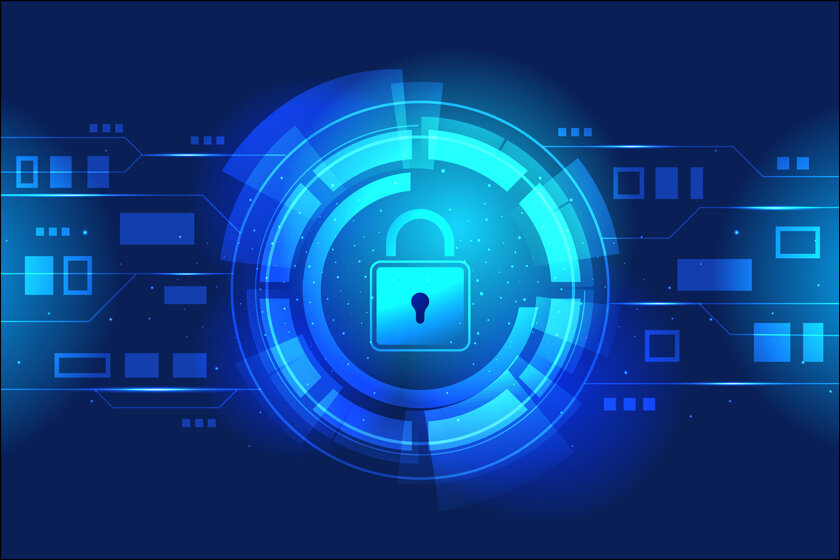
Limitations of Traditional Threat Detection Methods
Signature-based and rule-based detection methods, which match known malware signatures or specific behavior patterns, have significant limitations. They struggle to keep up with evolving and unknown threats, such as zero-day exploits and polymorphic malware. In addition, they have high false positive rates and do not stay effective for long without regular updates, exposing the system to security risks.
Applications of Machine Learning in Threat Detection
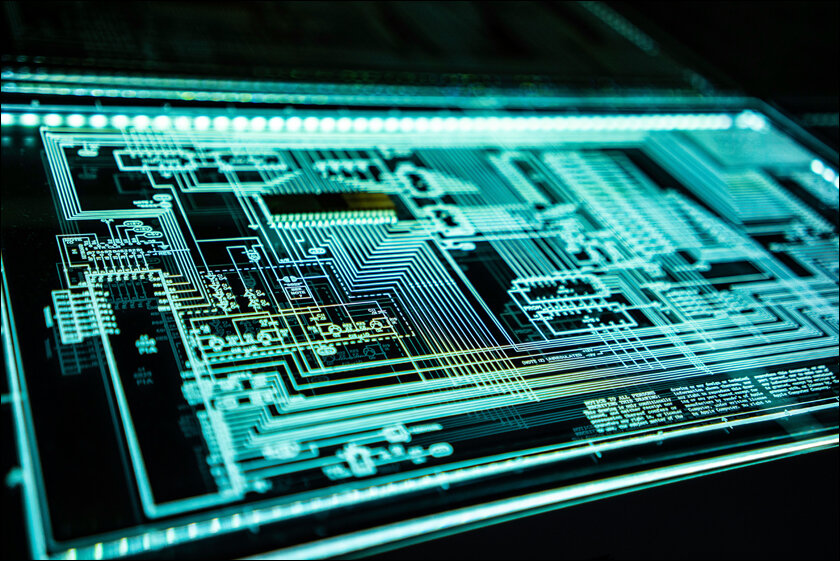
Machine learning algorithms enable systems to learn and improve from experience; systems do not need to be explicitly programmed.
- Malware Detection: ML algorithms detect anomalies in code behavior, which could mean new or modified malware; thus, zero-day attacks can be detected more efficiently than before.
- Network Traffic Analysis: ML algorithms can identify unusual traffic patterns, like increased data transfer on non-standard ports or excessive data transfers, which might indicate a DDoS attack or unauthorized data exfiltration.
- User Behavior Analytics: ML algorithms can learn normal user behaviors and alert security teams to anomalous activities. For instance, if a user accesses a large amount of sensitive information or logs in from a location that deviates from the normal pattern, the system can detect these events and may flag these.
- Predictive Analytics: By analyzing past cases and current trends, ML algorithms can predict likely future attacks, allowing organizations to fortify their defenses against such possible threats.
Advantages of AI-Powered Threat Detection
- Enhanced Threat Detection Capabilities through accuracy and speed
- Data Security Improvements through the identification and mitigation of threats
- Providing Real-Time Response to Cyber Threats, thereby decreasing the possible damage
- Scalability and Adaptability to emerging cyber threats
- Automating Security Processes by allowing machines to perform routine tasks and humans to make intelligent decisions
Challenges and Limitations of AI-Powered Threat Detection
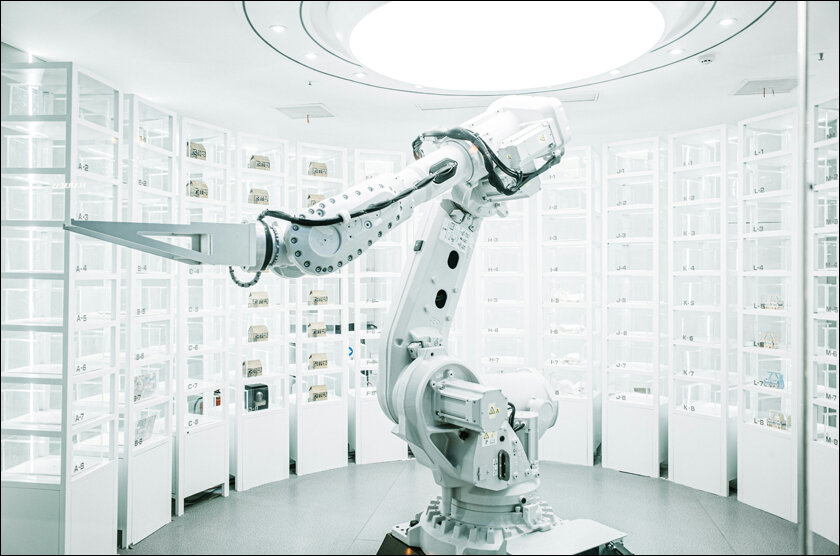
- Data Quality and Availability: Machine learning models depend on high-quality datasets for accurate threat detection.
- Interpretability and Transparency: The “black box” feature of common AI algorithms is an obstacle to cybersecurity professionals because they are unable to comprehend the reason behind threat detection.
- Integration and Deployment: Integrating AI into the existing cybersecurity infrastructure can be difficult and resource-consuming.
- Vulnerabilities in AI Algorithms: Adversaries may use tactics like adversarial ML to trick AI systems.
- Overcoming Bias in Machine Learning: It is important that training data is bias-free and representative to create fair and reliable AI-driven cybersecurity measures.
Conclusion
AI and ML are revolutionizing cybersecurity by increasing the detection and response speed, continuously evolving to avoid new threats, and becoming a driving force in creating secure digital platforms. As cyber threats evolve, the role of AI in cybersecurity will keep growing, which underscores the fact that one must accept the rise of these technologies to ensure the security of digital assets and data.
In case you missed:
- Securing the Digital Transformation Journey: Cybersecurity Pitfalls to Avoid
- Top 5 Cloud Security Threats and How to Combat Them
- Safeguarding Remote Workforce: Data Protection Measures
- The Importance of Cyber Hygiene: Ten Common Practices for Users
- Incident Response and Disaster Recovery: Preparing for the Worst-Case Scenario
- The Human Element: Why Security Awareness Training is Critical for Every Organization
- Common Data Protection Mistakes Businesses Must Avoid
- Understanding Phishing Attacks
- Data Classification and Risk Assessment: Foundations for Effective Data Protection
- Compliance and Data Protection: Navigating Complex Regulatory Landscapes