Artificial General Intelligence might seem like a pipe dream to many but to the engineering community the idea that AI might go beyond what it was programmed for is both a prologue to utopia and a premonition to dystopia, writes Nigel Pereira.
It’s been a crazy month for the scientific community with a researcher at DeepMind claiming “the game is over” in reference to the quest for Artificial General Intelligence (AGI), only to be followed by a Google engineer who has since been suspended for breaking his NDA and claiming Google’s AI is sentient. To the average person, these headlines signify a major technological leap with the expectation that we will soon see AI systems like J.A.R.V.I.S, Tony Stark’s AI Assistant from the Iron Man movies. Unfortunately, however, further research into the topic estimates we still have a considerable way to go.
Artificial Narrow Intelligence
Now there’s no denying that we have come leaps and bounds ahead of where we were ten years ago in regard to language processing which is evident from the leaked conversation with Google’s Lamda that got an engineer suspended. General Intelligence, however, is a lot more than an intelligent conversation, it involves common sense and awareness on a much greater scale. For example, a human whose job it is to deliver your groceries could quite possibly recommend a movie, a show, or even a restaurant if you asked him to. A neural network trained for Netflix, on the other hand, wouldn’t be able to help you with your groceries.
This is where the distinction between Artificial Narrow Intelligence (ANI) and AGI comes into the picture. While ANI is capable of outperforming humans at a number of single-minded tasks like board games, data processing, and even crawling websites, AGI is another thing altogether. ANI is the only kind of AI that humans have been able to develop so far, and this includes everything from Siri to Face ID, IBM Watson, and even DeepMind. To qualify as an AGI would require a neural network that can learn new tasks that are completely different from whatever it was trained to do.
Machine Learning Algorithms
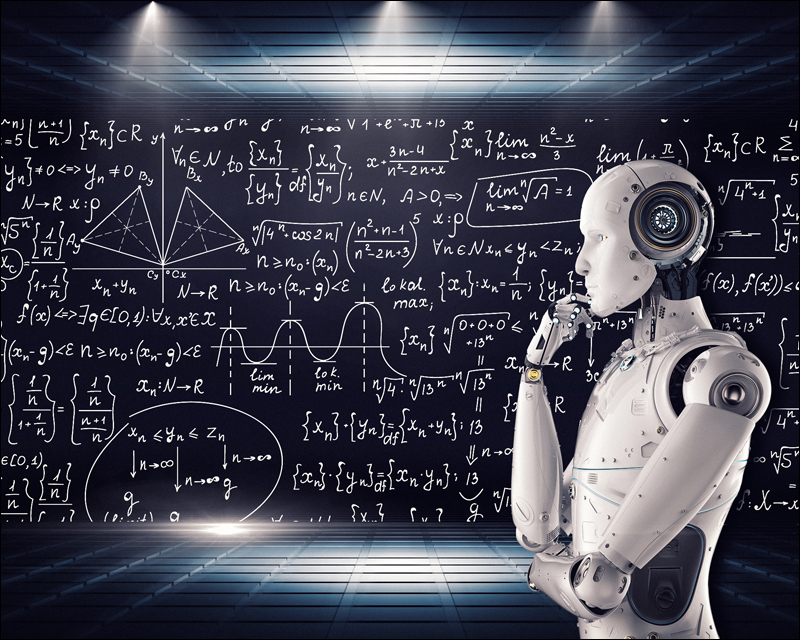
As opposed to traditional algorithms which were basically a set of instructions to be followed like a recipe, machine learning algorithms took things a step further by allowing machines to learn from their mistakes. For example, a machine learning algorithm at Google’s X-Lab learned to identify cats in YouTube videos without being told what a cat was. This is because machine learning algorithms excel at pattern recognition when fed vast amounts of data, so when it was fed about 1,00,000 YouTube videos, it learned to recognize cats with a 74.8% accuracy.
This rate of accuracy keeps going up as the machine learning algorithm keeps ingesting data and learning from previous mistakes, which is the crucial difference from traditional algorithms. This kind of self “optimizing” or fine-tuning a specific task like facial recognition or natural language processing is still a far cry from human-like intelligence. While it is true that computers are no longer dumb machines and can learn for themselves, they can’t apply that knowledge to a completely new task successfully. For example, while Siri or Alexa can recommend a movie for you to watch, neither of them can watch a film and reliably tell you what’s going on.
DeepMind’s Gato
Earlier in May this year, Google’s DeepMind unveiled its latest Deep Learning Transformer model called Gato which can perform 604 different unrelated tasks including stacking blocks with a robotic arm, playing video games, captioning images, and chatting with people. This is a big jump from DeepMind’s previous multi-function AI called Impala which could do about 30 different tasks. Gato achieves this by using a token system that decides whether its output should be in the form of voice, text, button presses, torque twists of a robotic arm, or other functions. While there are a lot of skeptics about how this moves us closer to AGI, there’s no denying this is a step ahead of ANI.
As for the now-infamous tweet saying the game is over and it’s just a matter of scaling up this model to achieve true AGI, that needs to be taken with a grain of salt. Packaging a lot of different ANI models together and then scaling them up isn’t magically going to create general intelligence. Mike Cook of the Knives and Paintbrushes research collective pointed out to TechCrunch’s Kyle wiggers that while it might seem that Gato can also make a cup of tea and learn 50 new tasks, that isn’t the case. Others have called Gato an illusionist capable of 600 different tricks.
General Intelligence
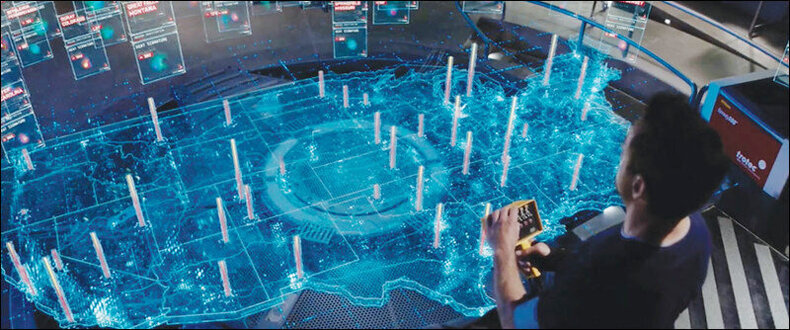
While some argue that general intelligence should be on-par with humans, others argue that humans are highly specialized and a human that’s a good driver may not necessarily be a good cook. If we actually do manage to replicate a human brain digitally, do we know the difference between what makes one an astronaut and what makes one a serial killer?
Since we’re still a long way away from figuring out our own intelligence, I think it’s satisfying to say we have progressed from ANI and now have AI systems that can at least mimic humans at hundreds of different tasks.
In case you missed:
- AI Uncovers Thousands of Potential Psychedelic Compounds!
- NVIDIA’s Isaac GR00T N1: From Lab Prototype to Real-World Robot Brain
- CES 2025: NVIDIA’s Cosmos Just Gave Robots a ‘ChatGPT Moment’!
- Slaughterbots: Robot Warriors in the Indian Armed Forces!
- Training AI for Pennies on the Dollar: Are DeepSeek’s Costs Being Undersold?
- Scientists gave a mushroom robotic legs and the results may frighten you
- So AI can get bored, “suffer,” and even commit suicide?
- China launches world’s first AI-powered underwater data centre!
- Lab-Grown Brain Thinks It’s a Butterfly: Proof We’re in a Simulation?
- AI in 2025: The Year Machines Got a Little Too Smart (But Not Smart Enough)
1 Comment
Good