When we examine ML vs. DL vs. AI, the nuances and opportunities become clear, Dinesh clarifies..
Artificial Intelligence (AI) fundamentally involves imbuing machines with human-like intelligence through predefined rules or algorithms. The term comprises “Artificial,” indicating human-made or non-natural entities, and “Intelligence,” denoting the capacity to comprehend and think logically. Another perspective defines AI as the discipline centered on instructing machines, particularly computers, to emulate human brain functions and cognitive processes.
The core pillars of AI revolve around three essential skills: learning, reasoning, and self-correction. This triad emphasizes the pursuit of optimal efficiency, underscoring AI’s goal to equip machines with the ability to acquire knowledge, make logical deductions, and autonomously rectify errors.
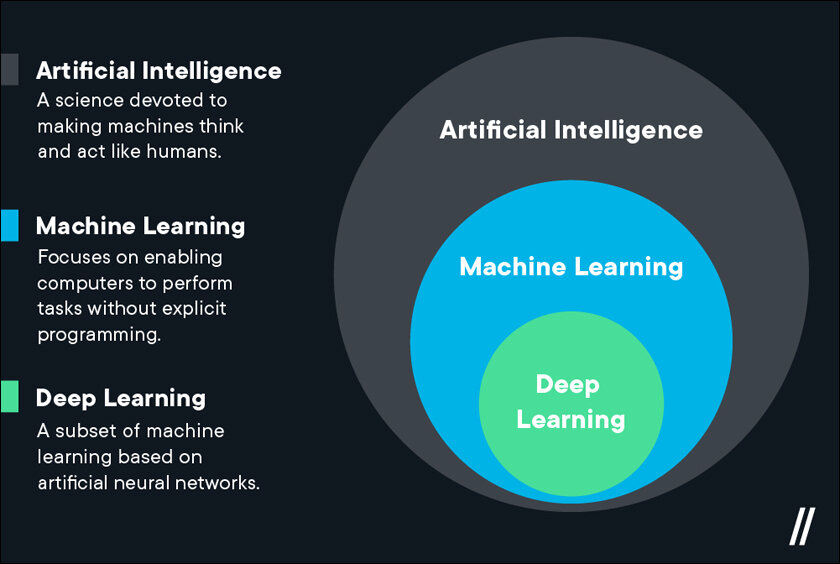
Machine Learning:
Machine Learning (ML) is essentially an investigative process empowering a system, typically a computer, to autonomously learn and enhance its performance based on experiences, free from explicit programming. Positioned as an application within the broader scope of Artificial Intelligence (AI), ML concentrates on crafting programs capable of accessing and utilizing data independently. This systematic approach involves observing data, recognizing emerging patterns, and leveraging insights from examples to refine decision-making processes. The primary objective of ML is to facilitate systems in self-directed learning, enabling them to evolve and improve through experiences, all without requiring direct human intervention or guidance.
Deep Learning:
Deep Learning (DL) serves as a subset within the expansive domain of Machine Learning, harnessing Neural Networks-similar to the neurons in the human brain-to replicate brain-like functionalities. DL algorithms delve into intricate patterns of information processing, mirroring the cognitive behavior observed in the human brain. This approach enables DL to discern and categorize information akin to the human brain’s pattern recognition. Notably, DL operates on more extensive datasets than traditional Machine Learning, and its predictive capabilities are autonomously administered by machines, emphasizing its capacity for sophisticated self-learning and decision-making processes.
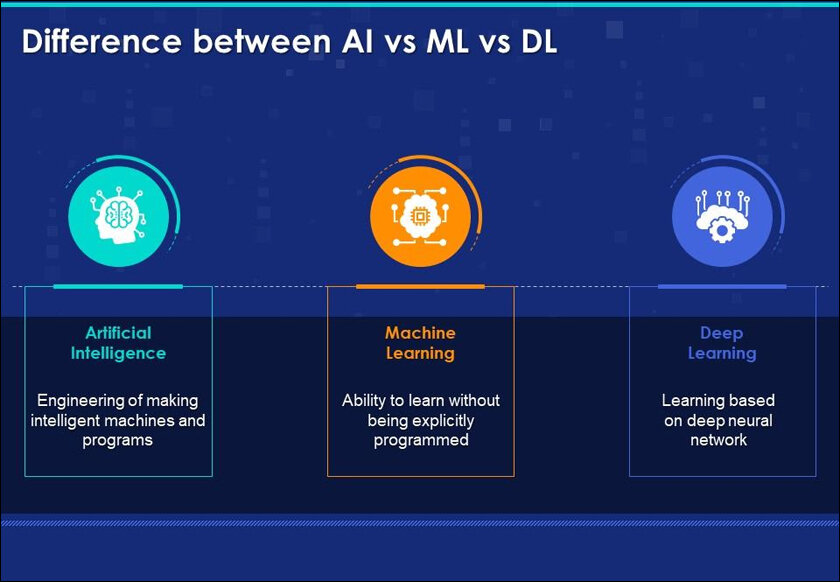
The following table lists the distinctions between deep learning, machine learning, and artificial intelligence:
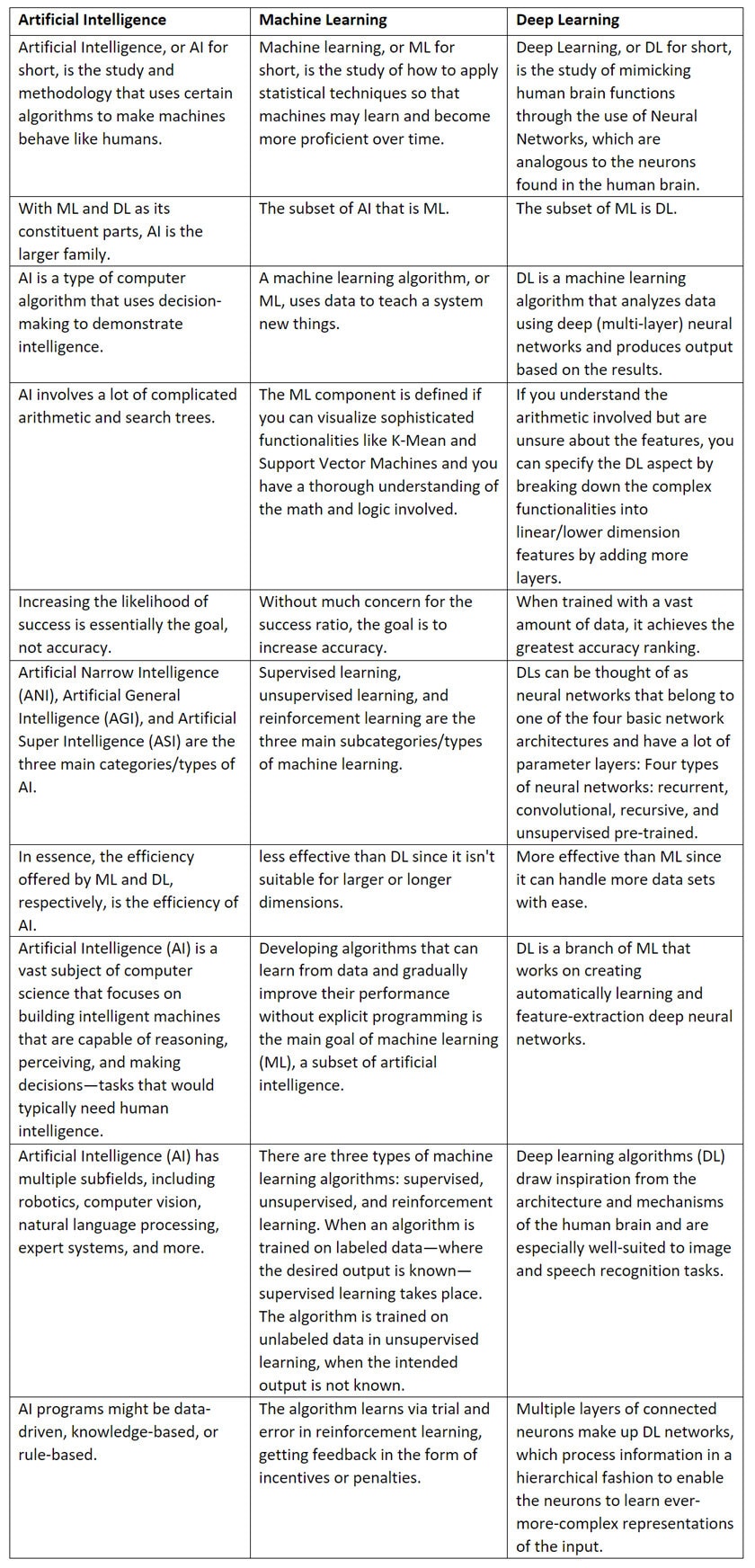
Examples of AI, Machine Learning, and Deep Learning
The creation of computer systems that are capable of carrying out activities that ordinarily require human intelligence is known as artificial intelligence, or AI. Some examples of AI include:
Applications of AI are widely available in a wide range of industries. Here are a few typical instances:
Speech recognition – Deep learning algorithms are used by speech recognition systems to identify and categorize audio and images. Numerous applications, including security systems, medical imaging, and self-driving automobiles, use these technologies.
Personalized recommendations – AI algorithms are used by e-commerce websites and streaming services such as Amazon and Netflix to examine the browsing and viewing histories of their users and suggest products and content that they are likely to find interesting.
Predictive maintenance – By analyzing data from sensors and other sources, AI-powered predictive maintenance systems can forecast when equipment is likely to break, which helps to save maintenance expenses and downtime.
Medical diagnosis – AI-driven medical diagnosis systems examine x-rays and additional patient data to assist physicians in developing more precise diagnosis and treatment regimens.
Autonomous vehicles – Autonomous vehicles, including self-driving automobiles, employ artificial intelligence (AI) algorithms and sensors to assess their surroundings and determine variables such as direction, speed, and other aspects.
Predictive analytics – AI is used to evaluate vast volumes of data to forecast future events, including disease outbreaks or consumer behavior, in sectors like marketing and healthcare.
Game-playing AI – By evaluating game data and forecasting move outcomes, artificial intelligence (AI) programs have been built to play games like poker, go, and chess at a superhuman level.
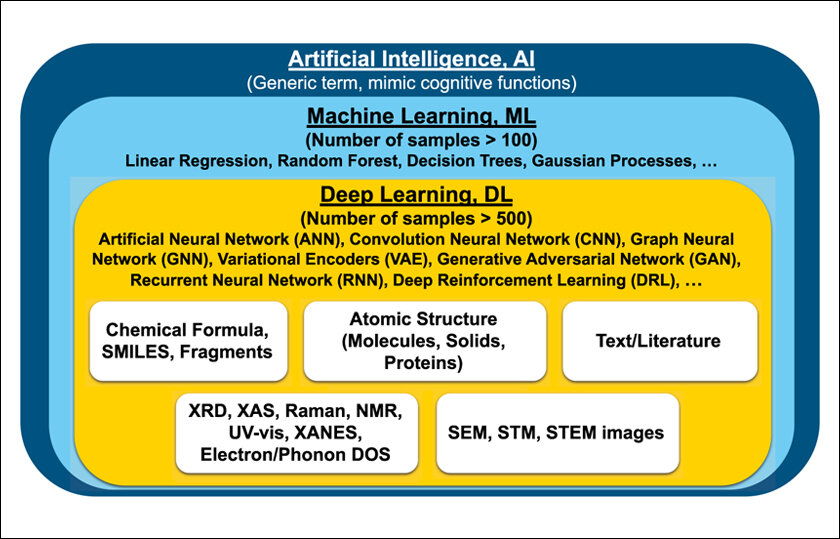
A branch of artificial intelligence known as machine learning (ML) uses statistical models and algorithms to let computers “learn” from data and get better over time without needing to be explicitly programmed to do so. Here are a few machine learning examples:
Image recognition – Image recognition systems identify photos according to their contents using machine learning methods. Numerous applications, including security systems, medical imaging, and self-driving automobiles, use these technologies.
Speech recognition – Speech recognition systems transcribe spoken language and identify the words using machine learning techniques. In addition to contact centers and other applications, these systems are used in virtual assistants like Siri and Alexa.
Natural language processing (NLP) – NLP systems use machine learning algorithms to comprehend and produce human language. Virtual assistants, Chatbots, and other applications involving natural language interactions employ these technologies.
Recommendation systems – Recommendation systems examine consumer data and suggest goods and services based on likelihood of interest using machine learning algorithms. Online stores, streaming services, and other apps leverage these technologies.
Sentiment analysis – Sentiment analysis systems utilize machine learning algorithms to categorize voice or text as positive, negative, or neutral. These systems are employed for various purposes, including social media monitoring.
Spam filters in email – Machine learning algorithms examine email information and content to detect and mark communications that might be spam.
Credit risk assessment – Financial organizations utilize machine learning (ML) algorithms to evaluate loan applicants’ credit risk by examining information including their income, work history, and credit score.
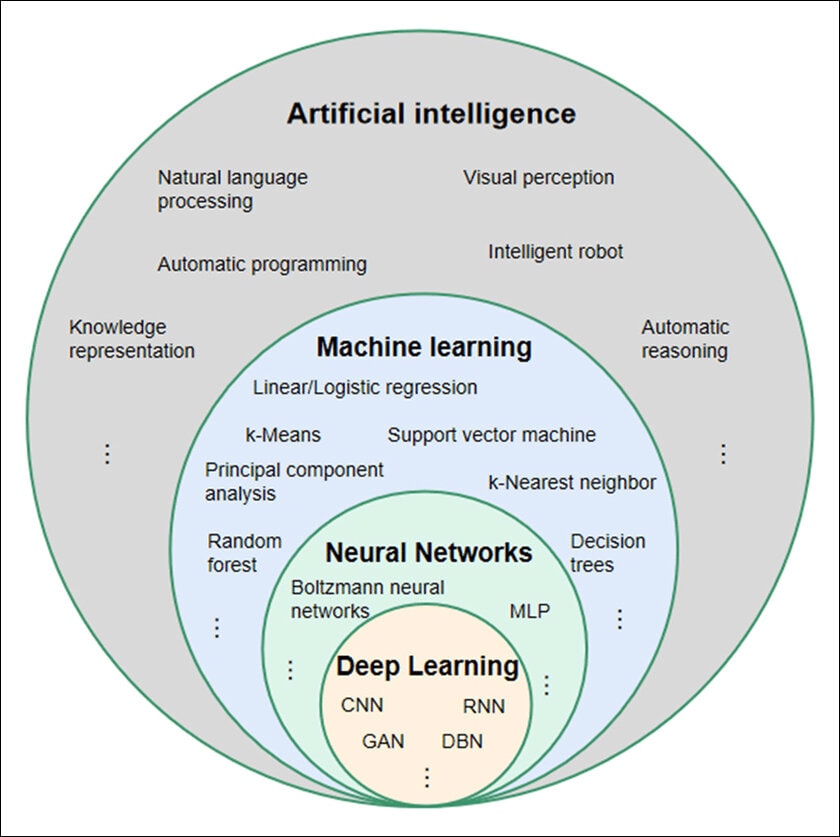
Artificial neural networks with numerous layers are used in deep learning, a type of machine learning, to learn and make judgments. Here are a few Deep Learning examples:
Image and video recognition – Systems for recognizing images and videos classify and analyze visual data using deep learning algorithms. Security systems, medical imaging, and self-driving automobiles all use these systems.
Generative models – Using deep learning algorithms and pre-existing data, generic models produce new content. These technologies are used in applications like text, video, and image production.
Autonomous vehicles – In order to evaluate sensor data and determine parameters like speed and direction, deep learning algorithms are employed in self-driving automobiles and other autonomous vehicles.
Image classification – For example, facial recognition in photographs or item identification in images for an e-commerce website are tasks that Deep Learning algorithms are employed for.
Speech recognition – Voice-activated interfaces and dictation software are made possible by the application of Deep Learning algorithms to translate spoken words into text.
Recommender systems – Recommendation systems employ deep learning algorithms to generate customized suggestions based on user behavior and preferences.
Fraud detection – In financial transactions, DL algorithms are used to identify behavioral patterns that point to fraud, like odd spending habits or transactions from strange places.
Game-playing AI – Artificial intelligence (AI) that can play games at a superhuman level has been created using deep learning algorithms. One example of this is the AlphaGo AI, which won the game of Go against the world champion.
Conclusion:
When we examine ML vs. DL vs. AI, the nuances and opportunities become clear. Get in-depth knowledge and practical experience to fully utilize these game-changing technologies and maintain your position at the forefront of innovation. I hope we’ve covered every facet of the three most widely used technologies and how they vary from one another.
If you have any queries in the comments section, we’ll consider them and respond as soon as possible with the answers.
In case you missed:
- Unveiling the Depths of Intelligence: A Journey into the World of Deep Learning
- An Overview of Machine Learning
- The Symbiosis of Medicine and Machines: Pioneering Technological Transformations in Healthcare
- Innovative Careers Shaping the Future: A Glimpse into Tech, Sustainability, and Cybersecurity
- The Role of AI in Driving Digital Transformation
- Navigating the Future of Healthcare: Ethical Considerations, Technological Trends, and Challenges in Integration
- The Automotive Industry’s Response to AI and E-Vehicles: Traveling Forward
- How Improved Illness Detection Might Be Aided by Extended Reality
- What’s the Difference Between Programming and Coding, Anyway?
- Technology Will Not Eliminate Your Work, but Someone Who Understands Technology Better than You May