Shadow AI is Information assimilation that operates in the background, often without consent or knowledge of the users.
In an apparent effort to find the right terminology for what the world perceives as ‘Artificial Intelligence,’ it is anything but artificial or intelligent. It is more accurately described as Assimilated, Acquired, Assorted, and Amalgamated Information. While this combination of words may not sound as appealing, it reflects the true nature of what is being discussed.
With the myriad of benefits, the series of articles this month would focus on the looming shadow of shadow AI.
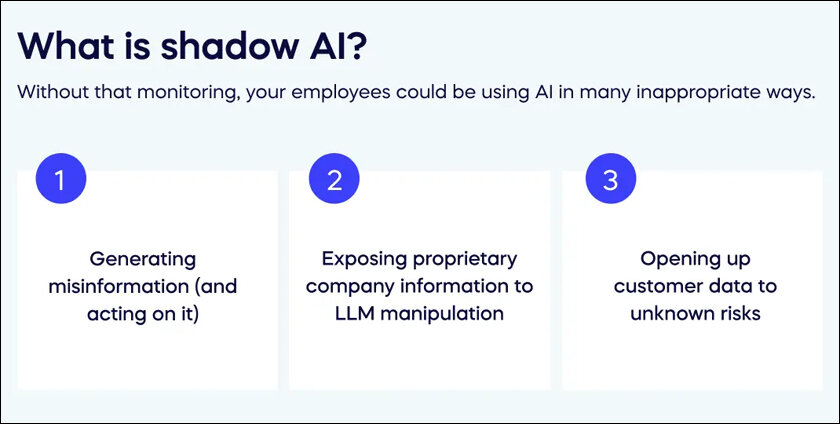
What is Shadow AI?
It is Information assimilation that operates in the background, often without consent or knowledge of the users. While the intent may be to foster transparency, accountability, the quest to assimilate information leads to an autonomous, opaque arena sans privacy, ethics or explicit accountability.
The quest to collect, align, and leverage data to respond to queries creates a competitive environment that often lacks accountability. A simple example could be the question of how many copies and caches of information are necessary to compile an accurate and comprehensive set of data. Additionally, there may be multiple versions of the same information, raising questions about their accuracy and consistency with references. The lack of accountability in this process can lead to a sense of helplessness and frustration among people who care about the integrity and accuracy of the information.
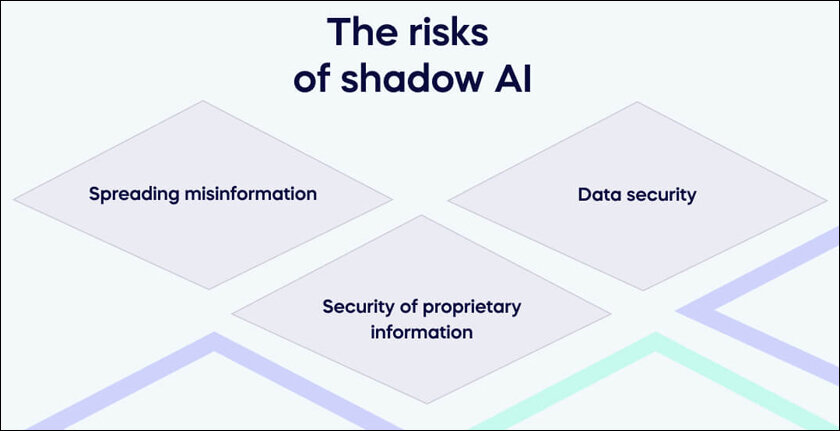
Bias amplified
While the data collected, processed and used creates potential privacy violations, the biggest issue is the creation of Algorithmic Bias. This can happen when the data used to train the algorithm is biased, or when the algorithm itself is designed in a way that is biased. The data driven and training nature perpetuates and amplifies the information led by what is being asked. This creates lack of control and undermines reality and agency. Algorithmic Bias. It could be biased in a way that is unfair or discriminatory to a particular group of people.
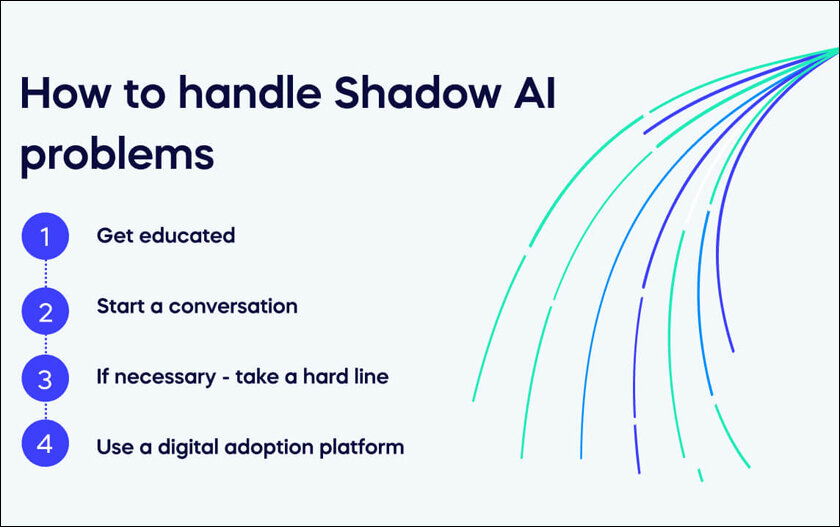
What are the ways to deal with it?
Besides verbose legal jargon privacy statements, it is essential to provide users with clear control over how their data is collected, processed, and used. Taking screenshots or photos from another camera, if screenshots are disabled, is an example of a necessary measure to ensure user control.
However, it is not sufficient for these measures to be merely a formality to avoid legal complications. They need to be meaningful and foolproof to effectively protect users’ privacy.
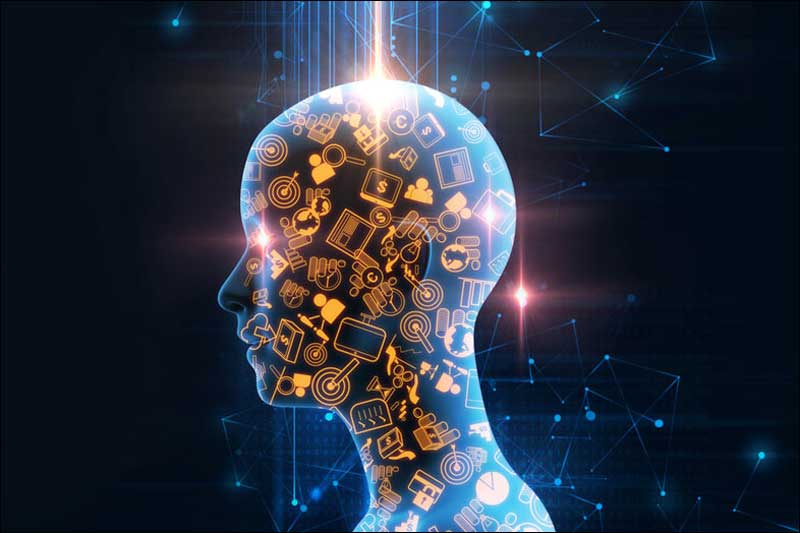
What is the practical solution or course of action to move ahead?
Some of the key aspects for any data provider (including us who inadvertently post videos, pictures or words in feeds!)
1. Transparency: Shadow AI systems should be designed to be transparent and accountable, providing users with clear information about how their data is being collected, processed, and used.
2. User Control: Users should be given control over their data and the decisions made by shadow AI systems. This can be achieved through providing users with the ability to opt out of data collection, review and modify the decisions made by the system, and seek redress in case of harm.
3. Algorithmic Auditing: Shadow AI systems should be subject to regular algorithmic audits to identify and mitigate any biases or discriminatory outcomes.
4. Regulation: Governments and regulatory bodies should develop comprehensive regulations for shadow AI systems to ensure they are used in a responsible and ethical manner.
By implementing measures to ensure transparency, user control, algorithmic auditing, and regulation, there is hope that these risks and ensure that shadow AI is used in a responsible and ethical manner.
In case you missed:
- ‘Art’ificial Intelligence
- Convergence Digital and Real – Is It Good
- Wearable frenzy – Fit or Fad?
- Technology to End Sexual Violence
- no-code – Is that the next Technology ‘Magic Eraser’?
- Assisted Intelligence – Are Assistants a thing of the past?
- Virtual Assessments- The Future of Exams?
- A ‘share’ of the success!
- AI and Opening up the Banks
- What is AI going to cost us?