In the dynamic landscape of data analysis, understanding the nuances of descriptive, diagnostic, predictive, and prescriptive analysis is paramount.
Data permeates every sector, from retail to healthcare, fuelling an era of unprecedented analysis. Data analysis, the conduit from raw information to actionable insights, enhances decision-making and problem-solving. Not all data analysis is uniform; its application varies based on purpose and context. This article delves into the four primary types: descriptive, diagnostic, predictive, and prescriptive.
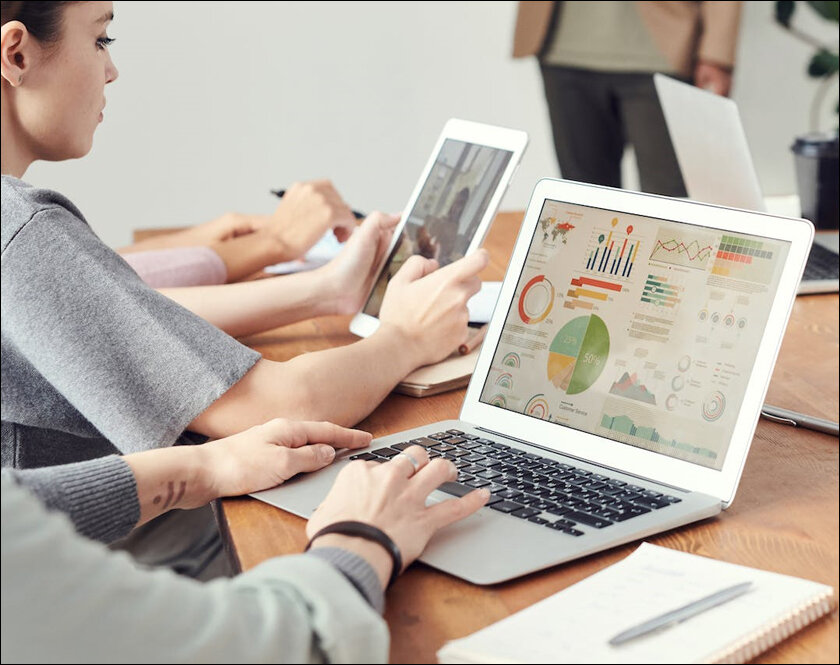
Concisely defining each type, exploring their mechanisms, and presenting real-world applications, we aim to elucidate their roles in diverse domains. By the conclusion, readers will possess a lucid comprehension of these methods, poised to wield them effectively.
Descriptive Analysis:
One important technique for exploring data is descriptive analysis, which focuses on describing historical events. It reveals important trends and patterns in historical data with an easy-to-use but effective methodology. It presents a historical perspective by revealing significant aspects of a dataset through thoughtful arrangement. Exploratory data analysis (EDA) is a crucial step in the descriptive analysis process that starts the investigation and understanding of data. EDA uses methods such as data visualization, data profiling, and summary statistics to find patterns and insights in the dataset.
Tools that are frequently used for descriptive analysis include:
Website owners may monitor and analyse important data, such as website traffic, user interactions, and conversion rates, with the help of Google Analytics. This tool is helpful in gaining insightful information and provides a thorough grasp of the operation of online platforms.
HubSpot offers comprehensive data analytics tools that let companies manage sales numbers, customer information, and marketing initiatives. This makes it easier to comprehend historical performance in its whole, which supports data-driven, well-informed decision-making processes.
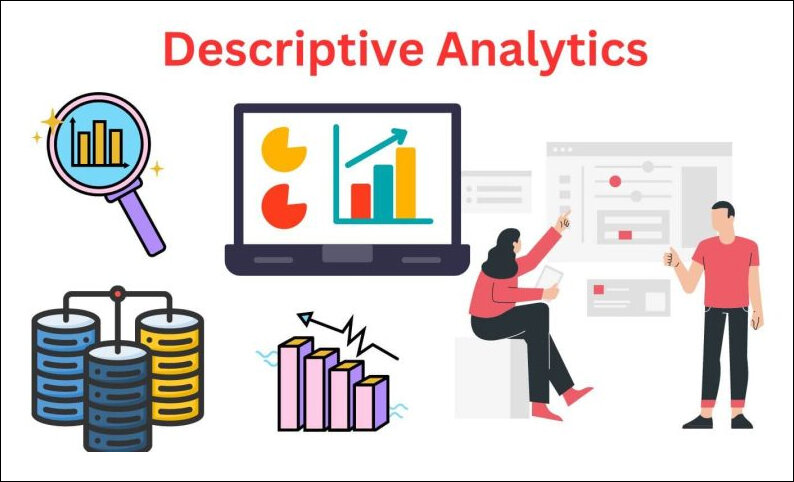
During the COVID-19 pandemic, statistical insights were deployed by governments and health organizations to track the transmission of the virus, monitor infection rates, and assess the efficacy of applied interventions. This is where descriptive analytics came into play.
Benefits of Descriptive Analysis:
- Summarizes and Simplifies Complex Data
- Identifies Patterns and Trends
- Communicates Insights Effectively
- Provides a Foundation for Further Analysis
Limitations of Descriptive Analysis:
- Descriptive Analysis Alone Does Not Explain Causality
- Lack of Predictive Power
- Limited Insights Beyond Summary Statistics
- Susceptible to Bias
Diagnostic Analysis:
Diagnostic analysis is a vital form of data analysis that investigates the reasons behind specific events or outcomes. Through an examination of patterns and relationships within the data, diagnostic analysis aims to reveal the fundamental causes and effects that contribute to particular phenomena.
Examples of Diagnostic Analysis:
A diagnostic method called “root cause analysis” can be used to determine the underlying cause of an issue or an incident. In order to help companies address the underlying cause of a problem instead of only addressing its symptoms, it entails evaluating data to identify the main factor that contributed to the problem.
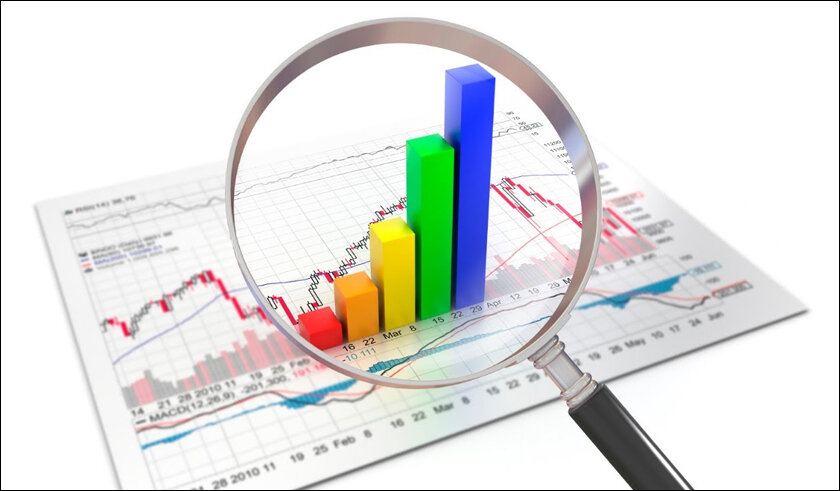
To determine the links between variables in a dataset, correlation analysis is used. It facilitates determining if and how much changes in one variable relate to changes in another. Organizations can learn how various elements impact one another by finding correlations.
Using segmentation analysis, a dataset is divided into discrete categories according to predetermined traits or standards. This helps businesses make focused decisions and develop unique strategies by enabling them to recognize trends and variances across various market groups.
Benefits of Diagnostic Analysis:
- Revealing Causes and Effects
- Informed Decision-Making
Limitations of Diagnostic Analysis:
- Lack of Future Predictability
- Contextual Factors
Predictive Analysis:
Predictive analysis stands as a potent data analysis technique, utilizing historical and current data to predict future events, trends, and outcomes. Through the application of diverse statistical and machine learning models, predictive analysis empowers organizations to make informed decisions and proactively respond to anticipated future scenarios.
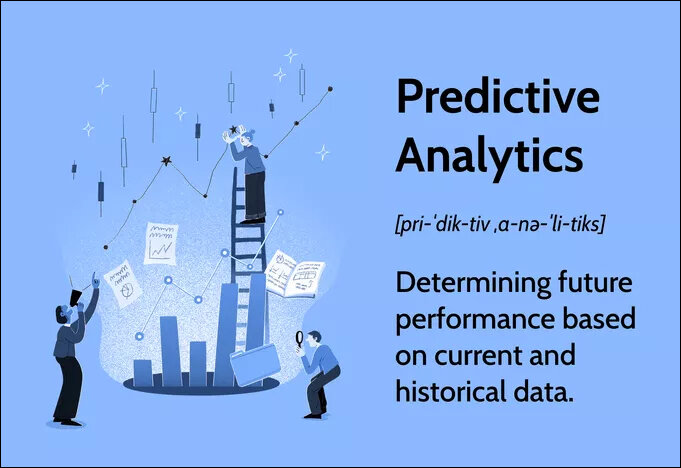
Examples of Predictive Analysis:
Predictive analysis uses machine learning methods extensively. These algorithms forecast new, unknown data by using patterns they have learned from past data. Regression models, which calculate values based on input variables, and classification models, such support vector machines or decision trees, are two examples.
A statistical method called regression analysis is used to find correlations between variables and forecast future results. Organizations can anticipate trends or estimate future values with its assistance in quantifying the influence of independent factors on a dependent variable.
Sentiment analysis is a technique that classifies and analyses subjective data from sources like surveys, social media, and consumer reviews using text data mining and natural language processing. Organizations are able to forecast market trends, customer satisfaction levels, and brand reputation by comprehending the emotion that is communicated.
Benefits of Predictive Analysis:
- Anticipating Future Trends
- Improved Decision-Making
Limitations of Predictive Analysis:
- Accuracy and Uncertainty
- Causality versus Correlation
Prescriptive Analysis:
Prescriptive analysis represents an advanced form of data analysis, surpassing mere predictions by recommending specific actions to attain desired goals or optimize a situation. Through the utilization of advanced algorithms and mathematical models, prescriptive analytics furnishes actionable insights that guide decision-making, ultimately maximizing outcomes.
Examples of Prescriptive Analysis:
Enhancement In prescriptive analysis, models are frequently utilized to determine the optimal course of action for intricate issues. These models provide the best possible resource allocation, scheduling, or production plans by taking into account a variety of restrictions and goals.
A graphical depiction of decision-making procedures, decision trees assist in choosing the optimal course of action by taking into account many circumstances and possible consequences. Taking into account variables and the probabilities that go along with them, prescriptive analysis using decision trees provides a framework for making well-informed judgments.
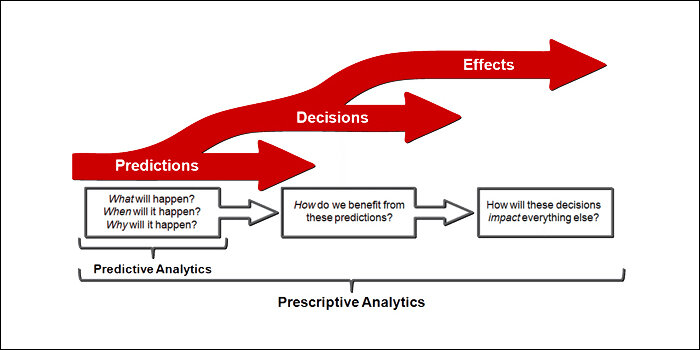
In order to test various scenarios and comprehend the behavior of real-world systems or processes, simulation models build virtual versions of them. Using simulation models for prescriptive analysis enables decision-makers to examine the effects of different actions and make well-informed decisions to maximize performance.
Benefits of Prescriptive Analysis:
- Actionable Solutions
- Optimized Results
Limitations of Prescriptive Analysis:
- Complex Data and Algorithms
- Costly Implementation
How to Apply Various Data Analysis Types:
Businesses looking to gain insights from their data now depend heavily on data analysis. With the variety of analytic methods available, it’s crucial to know which approach best fits your goals.
Selecting the data analysis type that best fits your goals is essential before starting any kind of data analysis project. Think about if you want diagnostic analysis to look into correlations and causes, descriptive analysis to describe and comprehend the data, predictive analysis to make future forecasts, or prescriptive analysis to recommend the best course of action. Making sure the kind of analysis you choose is in line with your goals will help you get the most out of your data in terms of insights.
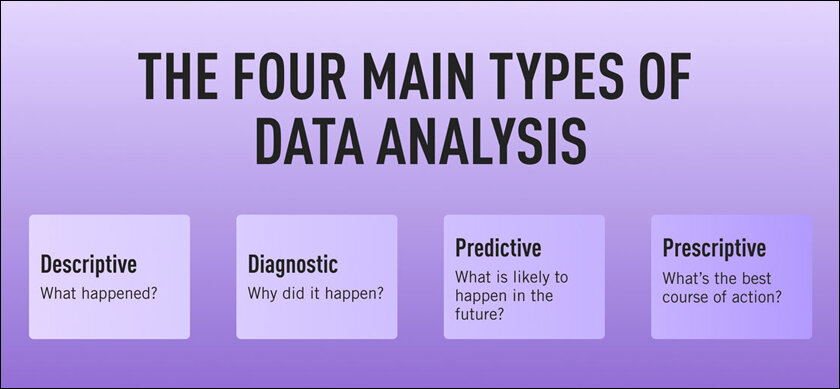
The steps to successfully apply each kind of data analysis are listed below:
In the realm of data analysis, a strategic progression unfolds, each step catering to specific objectives:
1. Descriptive Analysis:
- Approach: Utilize data visualization and summary statistics.
- Objective: Identify patterns, trends, and distributions for a comprehensive understanding.
2. Diagnostic Analysis:
- Approach: Delve deeper into data using statistical tests, hypothesis testing, and regression analysis.
- Objective: Investigate relationships, causes, and correlations, uncovering underlying root causes.
3. Predictive Analysis:
- Approach: Develop predictive models employing regression, time series analysis, or machine learning algorithms.
- Objective: Train models on historical data, validate performance, and make data-driven projections for future predictions.
4. Prescriptive Analysis:
- Approach: Employ optimization models, decision trees, or simulation models.
- Objective: Recommend optimal actions, considering constraints, objectives, and multiple variables, guiding decision-making for maximized outcomes, accounting for operational trade-offs.
In the dynamic landscape of data analysis, understanding the nuances of descriptive, diagnostic, predictive, and prescriptive analysis is paramount. Each type serves a distinct purpose, unravelling insights from historical, causal, predictive, and actionable perspectives. Google Analytics and HubSpot exemplify their applications, elucidating the role of data in diverse domains, from online platforms to global health crises. While descriptive analysis simplifies complexity, diagnostic analysis delves into causality. Predictive analysis anticipates trends, and prescriptive analysis guides optimized actions. Navigating these methodologies equips decision-makers to extract meaningful insights, fostering informed strategies in an era were data reigns supreme.
In case you missed:
- Distinguishing Deep Learning, Machine Learning, and Artificial Intelligence
- The Role of AI in Driving Digital Transformation
- Transforming India’s Organic Food Landscape: A Tech-Infused Revolution
- The Symbiosis of Medicine and Machines: Pioneering Technological Transformations in Healthcare
- An Overview of Machine Learning
- Innovative Careers Shaping the Future: A Glimpse into Tech, Sustainability, and Cybersecurity
- The Growth Of Apps For Mindfulness: How AI Can Help With Stress Management
- Unveiling the Depths of Intelligence: A Journey into the World of Deep Learning
- Why Choose a Smart Ring over a Fitness Tracker and What Do They Mean?
- The Automotive Industry’s Response to AI and E-Vehicles: Traveling Forward